Gen AI: Overhyped or Long-Term Revenue Generator?
Finding revenues amid challenges in adoption and packaging
Integrating generative AI offers significant opportunities and challenges for companies amid an extreme technology hype cycle. With AI spending down and many projects failing, platform and application companies can thrive by simplifying AI integration and packaging. Success depends on strategic pricing, market adoption, customer research, and leveraging competitive advantages to enhance adoption and revenue.
Request Access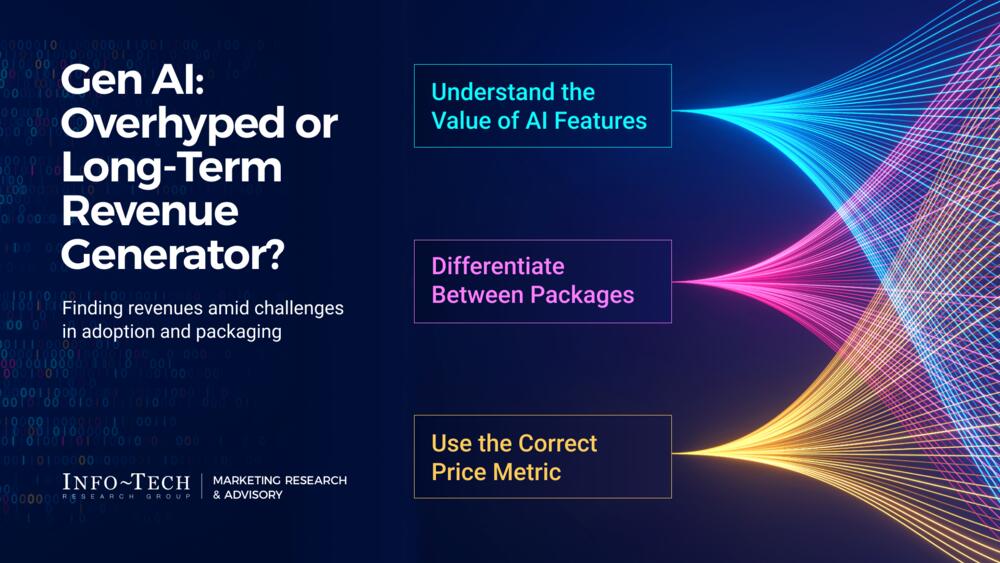
Included Research:
Name | Actions |
---|---|
Gen AI: Overhyped or Long-Term Revenue Generator?
|
Introduction
As software companies strive to innovate and deliver more value, integrating generative artificial intelligence (Gen AI) features and creating new packages and revenue streams presents unique opportunities and challenges. The timing of education and customers’ ability to understand the value and productivity gains while comprehending the risk and purchasing needs to be in sync for new revenue growth.
We are amid one of the most significant and sharpest technology hype cycles ever, comparable to Y2K and the cloud, and this is happening without a recession or macro-level event dampening the momentum. Every decade, the technology sector experiences a new overhyped cycle.
Gen AI statistics are unsurprising because technology overhype and revenues – i.e. purchases – operate on different timelines. History has shown that customer adoption of new innovations, which require risk and education, takes years of investment, not months.
- AI spending plans are down one-third year over year: Just 63% of global companies plan to increase AI spending in the next 12 months, down from 93% in 2023, according to Lucidworks’ second annual generative AI study.
- Nearly one in three Gen AI projects will be scrapped. Even as companies race to roll out generative AI tools to be more competitive and productive, several hurdles lead to pilots needing to be abandoned (Computerworld, 2024).
The early-stage Gen AI hyped technology has delivered underwhelming productivity results. The productivity paradox shows that technological advancements often outpace workforce productivity gains. This paradox arises from steep learning curves, the need for workforce development, and misalignment between technology and business processes. When this happens, anticipated revenue gains are often delayed. Deploying new technology requires updating systems to avoid overwhelming employees. We risk another productivity paradox with generative AI if we don’t rethink how we educate and help buyers achieve their productivity goals over reasonable time frames.
There will be winners, and ServiceNow is likely one of them. Per its July 2024 Earnings call, its AI-updated IT helpdesk solution now saves 45 minutes per avoided case and 30 minutes in the customer service realm. They are targeting 21,000 hours saved across all workflows, including the development/coding of non-complex scripts. We see this as the future of success vs. the build-your-own options or other mega-vendors like Salesforce that have cobbled together dozens of companies but never refactored the codebase.
With the above Gen AI hype and timing in mind, software businesses must keep up with the latest pricing and packaging trends and adopt a blend of technology, skilled expertise, and strategic foresight to stay ahead of the competition.
To successfully monetize AI features, software companies must understand their role within their products and differentiate them across various packages to cater to customer needs and budgets. Selecting the right pricing metric, whether user-based or usage-based, ensures transparency and fairness. Implementing best practices such as categorizing AI features, creating an attractive upsell path, and establishing a fair-use policy, along with thorough customer research and competitor analysis, can help set the optimal price point and enhance adoption and willingness to pay.
Status of Gen AI Pricing and Packaging One Year Later
The ongoing macro pressures on software companies and conservative growth forecasts from software management teams highlight current challenges. Renewed debates about Gen AI tools enhancing productivity and reducing seat growth for seat-based licensing models add to these pressures.
Despite these hurdles, AI innovations will drive incremental revenue through new Gen AI SKUs, increased pricing power on core services, and accelerated cloud migrations for AI access. However, full integration into off-the-shelf applications and tools will take time.
This comparison below highlights how both OpenAI and Microsoft leverage their unique strategies and competitive advantages to maximize revenue and meet customer needs in the Gen AI market.
|
OpenAI’s ChatGPT |
Microsoft's Azure OpenAI Service and Copilot |
---|---|---|
Package Differentiation |
Basic functionalities in entry-level packages; advanced features in premium tiers |
Gen AI monetization at the infrastructure level; basic integrations into 365 |
Model Variation |
GPT-3.5 was just replaced for the entry tier by GPT-4o mini, which aligns with the concepts of microLLM. ChatGPT also offers a small language model and multi-LLM architecture. ChatGPT, Free, Plus, Team, and Enterprise all include access GPT-4o mini. |
Enterprises can create APIs using OpenAI GPT models |
Monetization Strategy |
Converts millions to paid tier by offering better models and features in higher tiers |
One of the few companies with Gen AI monetization via Azure infrastructure |
Revenue Potential |
Maximizes revenue by aligning features with user needs |
Enables enterprises to build Gen AI apps with private data, enhancing value |
Competitive Advantage |
Provides access to less advanced, cost-effective models in lower tiers |
49% ownership in OpenAI, leveraging AI capabilities with Azure integration and 365. Very large ecosystem of developers and customers to sell into and upgrade. |
Product and marketing managers can improve profitability by understanding how to optimize price and packaging strategy:
- Implementing tailored price changes (versus across-the-board price actions) to help account for inflation exposure, customer willingness to pay, and product attribute changes.
- Accelerating ongoing pricing decision-making with a resolute cross-functional team ready to act quickly.
- Resetting discounting and promotion and revisiting service-level agreements.
An optimized price strategy is… |
Price strategy is not… |
---|---|
|
|
Integrating AI and Machine Learning in Pricing Strategies Marks a Significant Shift Toward More Dynamic and Informed Pricing Decisions
The trends in AI packaging and pricing for standalone products and those integrated with other solutions are evolving rapidly, driven by technological advancements and changing market demands. These trends reflect a significant shift toward embedding AI into broader software ecosystems, leveraging AI to enhance functionalities, and providing flexible pricing models to meet diverse customer needs.
AI Standalone Products |
AI Bundled Products |
---|---|
|
|
Understand the Value of AI Features
To successfully monetize AI features, it is essential to understand their role within the product by answering these five critical questions:
- What are the AI packaging trends for your market?
- What are your competitors doing?
- What is the value to the customer?
- How many customers will use this feature?
- How much are they willing to pay for it?
Tailor the monetization strategy to maximize revenue by aligning feature offerings with customer needs and payment readiness. By thoroughly understanding customer interest and price sensitivity, businesses can create compelling packages and pricing models that enhance adoption and profitability.
“We find that approximately 50% of SaaS companies have a Good/Better/Best packaging structure, and in our projects, we often see that Gen. AI features are leader features.”
Source: Simon-Kucher, 2024
To effectively monetize multiple generative AI features, divide them across good-better-best packages to create a compelling upsell path. Allocate features based on each package's target customer segment or use case. This approach ensures an attractive and clear land-and-expand packaging structure with a unique story for each package. By aligning features with specific customer needs and creating distinct value propositions, businesses can drive higher adoption and maximize revenue.
Differentiate Between Packages
Not all AI features need to be in a single package. Differentiating features between packages can cater to a broader range of customer needs and budgets. For example, basic functionalities could be in the entry-level package, while advanced features are reserved for premium tiers. Bundling all generative AI features into a single package or add-on is unnecessary to cater to a broader range of customer needs and budgets.
Implementing best practices like AI feature categorization helps identify the most valuable features to customers. Classifying features as leaders, fillers, add-ons, or killers based on customer interest and willingness to pay allows for strategic pricing and packaging decisions. Creating an attractive upsell path with a straightforward story for each package can enhance customer experience and drive sales.
Additionally, establishing a clear fair-use policy maintains a sustainable service for providers and users, ensuring that the AI features remain effective and valuable over time. This comprehensive approach will boost immediate sales and secure customer loyalty and market position overall.
Use the Correct Price Metric
A user-based fee for generative AI that guarantees fair usage and has fences to upsell heavy users is often very explainable and easy to implement.
Although usage-based pricing has become common in the SaaS industry, generative AI solutions vary considerably. Some use a user-based fee, while others charge by credits or per query.
Pricing metrics can be based on:
- Subscription – Per user per month
- Query Based – Credit limited or per query
- Consumption – Computing power, storage, data processing, and AI services
- Modular – Add-on AI features
- Custom – Negotiated based on enterprise scale and specific needs
The ideal price metric should be easy to explain, scalable with value, and implementable while ensuring billing transparency and fairness. Usage-based metrics are effective for landing and expanding customers, as they scale the price with the value experienced by the customer. They can serve as fencing mechanisms between packages.
However, some generative AI applications offer “unlimited” usage to promote frequent use. A fair-use policy is advisable to mitigate high usage risks leading to resource strains. This policy sets clear usage boundaries, ensuring sustainability for the provider and affordability for the user while maintaining an open and honest customer relationship.
Your Sales, RFP, and Contract Processes Are Longer and More Involved, Including Other Departments Outside of Just Business or IT Operations
Shadow IT, including SaaS and other IT products and contracts entered without IT’s input and awareness, already poses significant challenges. However, shadow AI can exponentially worsen the impact on your customers’ organizations.
AI contracts carry more significant potential risks due to using and disclosing intellectual property, personally identifiable information, confidential information, proprietary information, and other sensitive data. Your customers will be implementing more robust governance and oversight for AI contracts to mitigate these risks effectively, so we recommend looking at the governance and frameworks related to the acquisition process. Review Prepare to Negotiate Your Generative AI Vendor Contract and AI Contracting Primer: Update your contracts and contracting process to minimize your risk – Part 1.
Recommendations
To set the right optimized price for generative AI features and profit, follow these four critical elements to correctly price your product, give complete and accurate quotations, choose the terms of the sale, and select the payment method. Product and marketing managers can improve profitability by:
- Implementing tailored price changes (versus across-the-board price actions) to help account for inflation exposure, customer willingness to pay, and product attribute changes.
- Accelerating ongoing pricing decision-making with a dedicated cross-functional team ready to act quickly.
- Resetting discounting and promotion and revisiting service-level agreements.
- Reviewing our pricing blueprints Optimized Software Pricing in a Volatile Competitive Market and Priced to Win: Build an Optimized Pricing Strategy.
By following the above steps, businesses can set a price for various types of packaging that resonates with their target audience while trying to sustain a healthy profit margin.
Conclusion
Most software companies need a separate Gen AI SKU for monetization, and those with one are still waiting to see significant revenue. Moreover, many proofs of concept (POCs) need to transition to production, ROI for new use cases remains unclear, and modeling costs for consumption pricing is complex. As we navigate the “trough of disillusionment” phase, we must focus on:
- Developing clear monetization strategies: Innovate and create attractive pricing models for Gen AI offerings.
- Converting POCs to production: Strengthen support and resources to successfully transition from POCs to full-scale production.
- Demonstrating ROI: Develop robust case studies and success stories to illustrate Gen AI implementations' ROI.
- Simplifying consumption pricing: Develop straightforward and transparent pricing structures to simplify customer cost modeling.
By addressing these areas proactively, we can turn current challenges into opportunities, paving the way for the long-term success and appreciation of Gen AI technologies over the next five to ten years.
Other Related Publications and Deliverables
- “Key Pricing Trends In 2024: AI Conquers the Mainstream,” 7Learnings, 5 March 2024
- “Pricing Trends in 2024,” Academy 4 Pricing
- “Best Practices in Generative AI Packaging and Pricing,” Simon-Kucher, 4 March 2024
- “ServiceNow Reports Second Quarter 2024 Financial Results.” ServiceNow, 24 July 2024
- “Nearly One in Three GenAI Projects Will Be Scrapped, Computerworld, 30 July 2024
- “The State of AI in Early 2024: Gen AI Adoption Spikes and Starts to Generate Value,” McKinsey & Company, 30 May 2024
Info-Tech Articles and Blueprints on Pricing and Packaging
- Optimized Software Pricing in a Volatile Competitive Market
- Priced to Win: Build an Optimized Pricing Strategy
- Adopt a Structured Acquisition Process to Ensure Excellence in Gen AI Outcomes
- AI Contracting Primer: Update your contracts and contracting process to minimize your risk – Part 1
- Prepare to Negotiate Your Generative AI Vendor Contract